Salarybands
Empowering everyone to make better pay decisions. Salarybands is a web app that crowdsource compensation data for marginalized and underrepresented groups in tech.
Problem Background
Wage gap is one of the important measures for evaluating equity in the workplaces. A widespread demographic descrimination continues to be present in society irrespective of companies’ publicly acclaimed Diversity, Equity and Inclusion (DEI) commitments.
Research shows that certain minority groups experience persistent economic inequality due to large gaps in wages when compared with their counterparts. Thus, people from certain marginalized backgrounds based on gender, race, disability, and neurodevelopment backgrounds are more likely to be paid below the job market value.
There is an opportunity to democratize pay information by making salary information readily available to professionals to enable them to initiate fair and informed salary negotiations.
Research Insights
Using our network from forums and social media, we surveyed and interviewed different categories of prospective users from diverse backgrounds. From the survey received from over 262 survey respondents, the common themes were that:
- 71% believe that they must switch companies in order to be paid fairly.
- 73% prefer to work at companies that discloses pay information
- 79% expressed that they have been denied a raise because of their gender.
- 64% said that they were denied a raise due to their race.
- 74% said that they would leave their job if they found out that they were being underpaid.

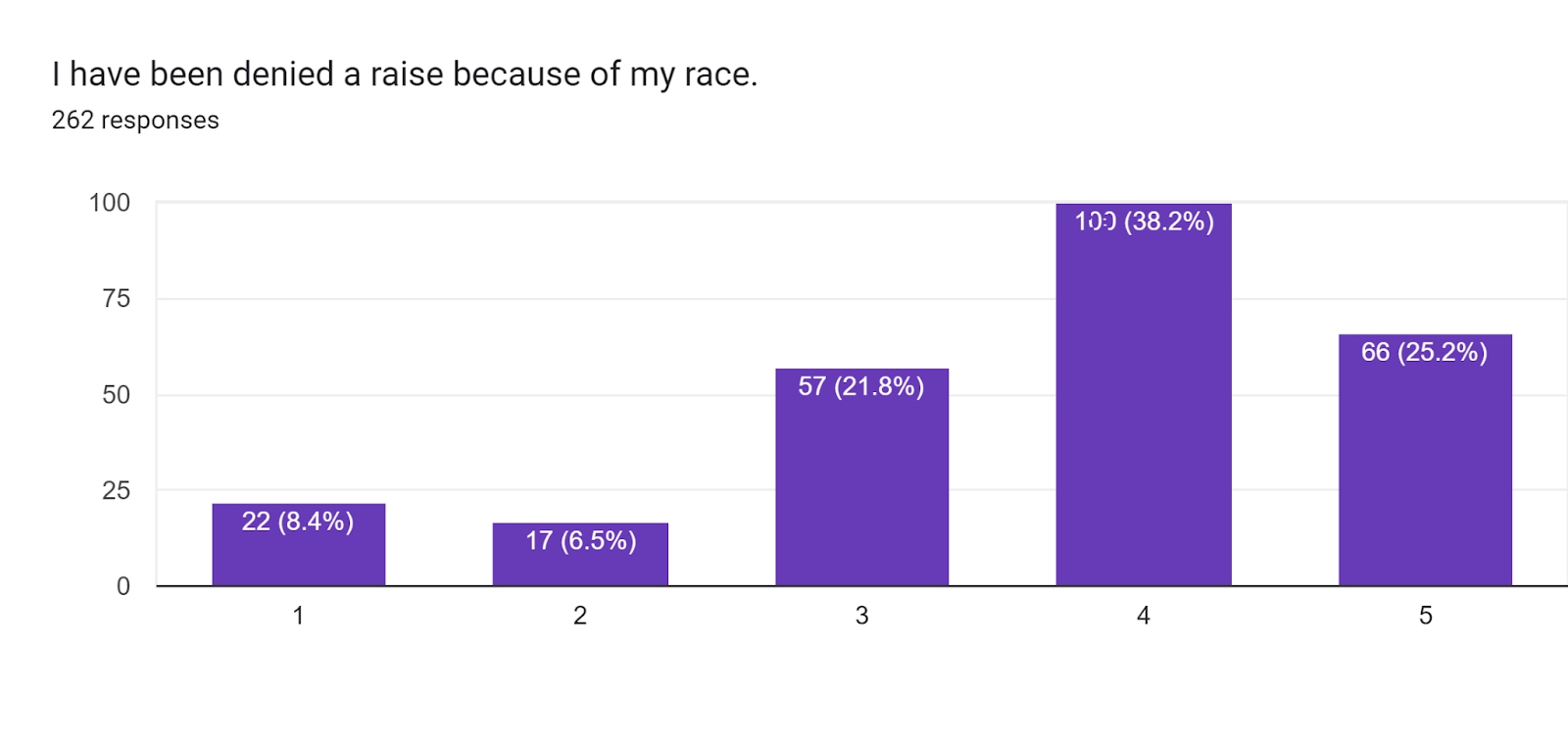

Our preliminary survey and interviews with job seekers and professionals revealed the gaps in salary transparency. The main issue was first-time job seekers found existing salary databases to be too generic and lacked detailed salary information that was focused on relevant demographics and data they cared about. Another problem was that some professionals found it daunting and unnerving to discuss topics about salary with their colleagues or company to assess pay equity because of their minority profiles. Other participants expressed that they lack confidence and an action plan on how best to negotiate their salaries.
Solution Explanation
Based on our target users’ pain points, we knew we wanted to work on a solution that provided the following benefits::
- Diverse Salary Database: Find specific and detailed salary data by incorporating advanced filters and demographic-based tags.
- Dependable and Accurate Data: Salaries published are verified for accuracy and correctness. We do all of this anonymously.
- Community Oriented: Leverage the success and advice of others who successfully negotiated their salaries to inform your career decisions.
Prioritization Framework
Using the insights gained from our user research, we leveraged the RICE framework to help us prioritize features that highlight the core values and goals of the solution.

Our learnings: The RICE scoring model helped the team to quickly evaluate and prioritize the value of the features that were planned for the sprints.
In our MVP, we initially decided to prioritize both the database view and the salary upload as the main features due to implementation complexity and time constraints.
Even though features such as salary advice and demographic filters were going to provide high value for our users, the PM made a decision to descope and deprioritize those features because of the high technical risk so that we can focus on the main features that will enhance the intuitiveness and user experience of the product.
After the main features were deployed into production, we revisited and reprioritized the two features that later shipped successfully.
Lo Fi & Hi Fi Mockups
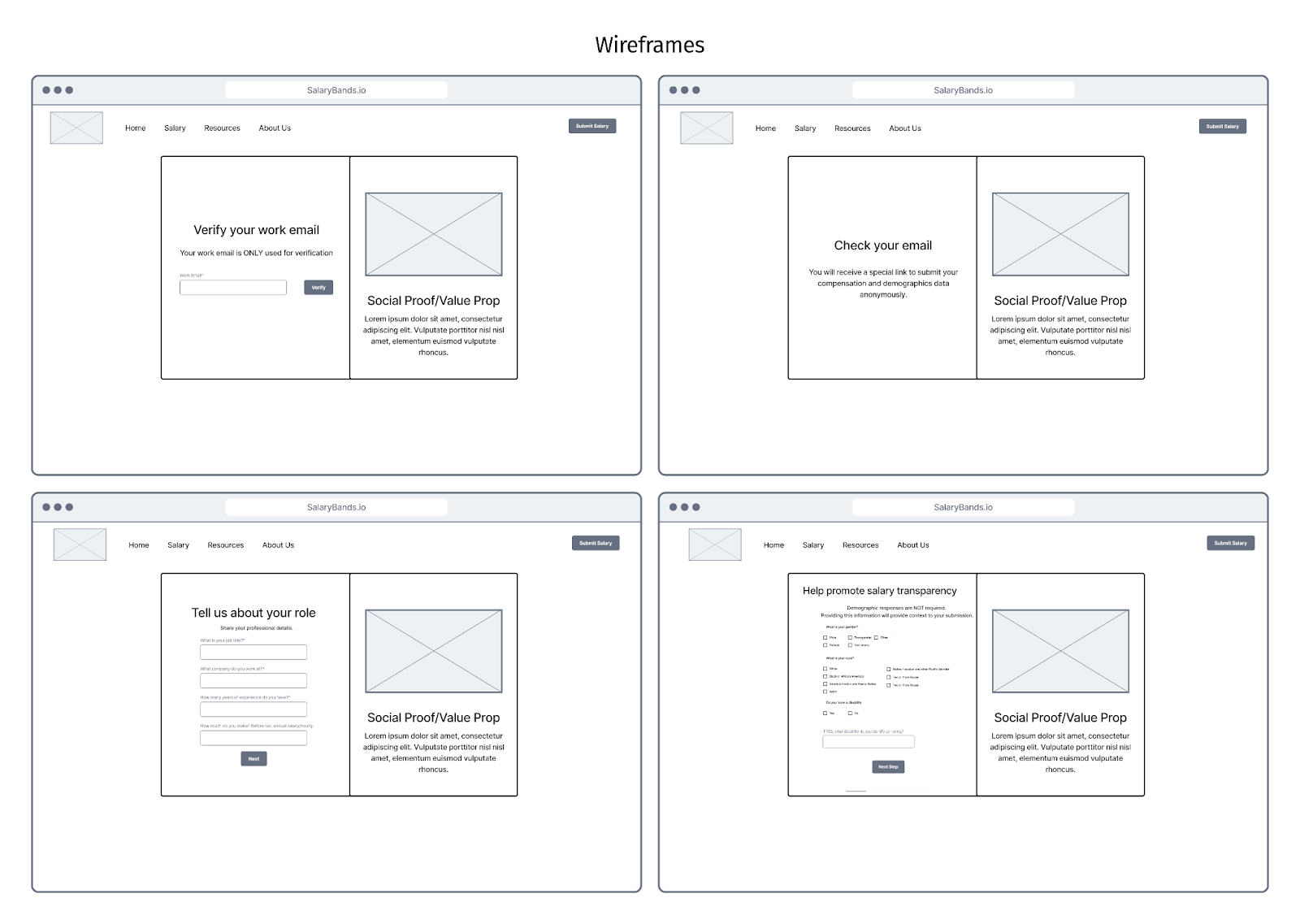

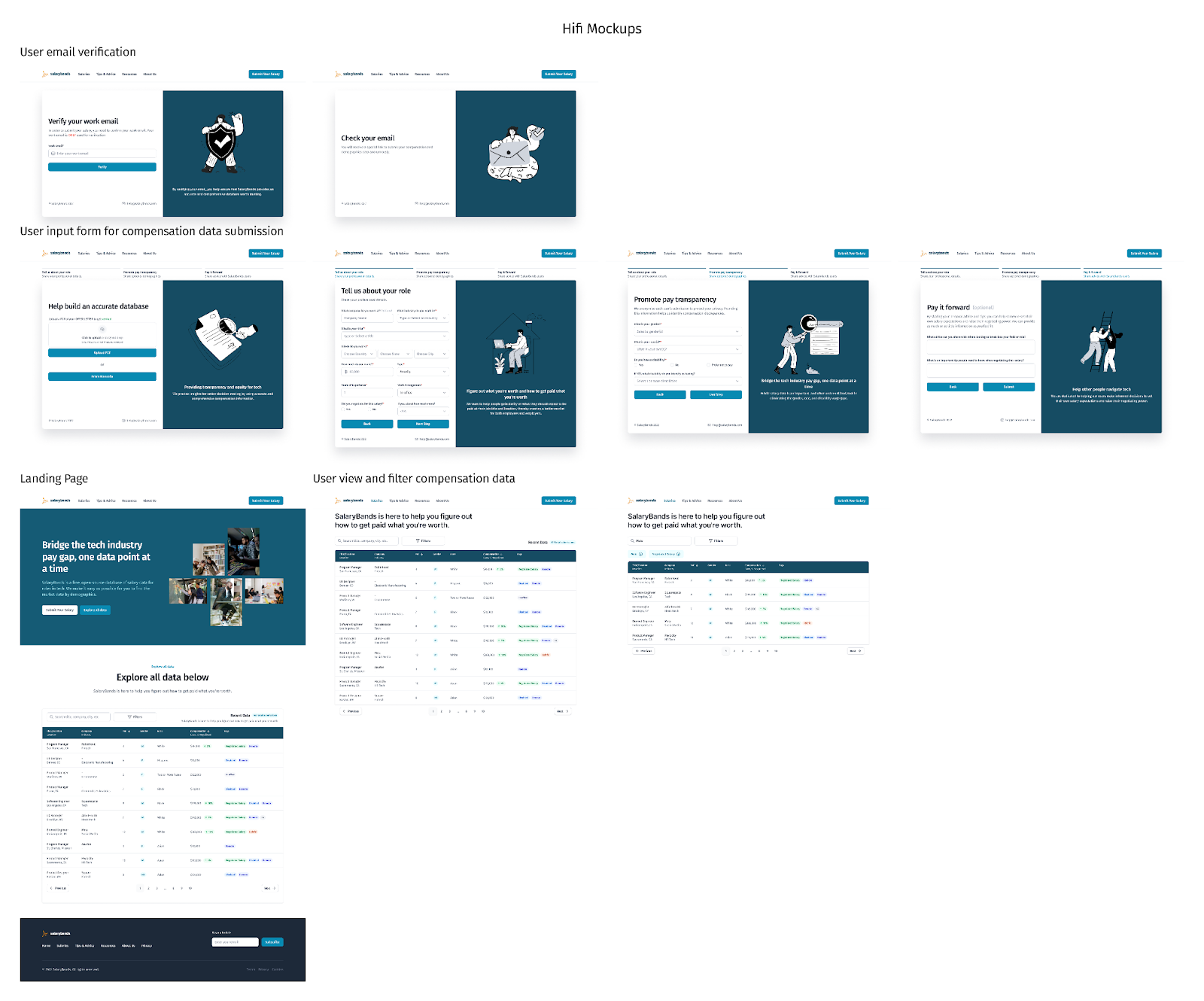
Iterative Design Learnings
After we showcased our prototype to the users again, we learned that the design resulted in 95% average task completion rate of submitting data. However, a couple users demonstrated challenges understanding what input fields were optional versus required. Therefore, we identified two opportunities to improve the design.
- Marking required and optional fields in input form.
- Include better error/destructive messages for required input fields.

Overall, users expressed that they would use this tool in the future to determine the market salary of their desired role.
Implementation Details
Technical implementation
We used Rest Api for the backend and fetched the data in the frontend. The backend is deployed on Render.com and the Frontend on Netlify.com.
About the stack, we used:
Backend:
- Language: Ruby
- Framework: Ruby on Rails
- Authentication: JWT
- Email server: Sendgrid
- Test: Minitest
Frontend:
- Languages: Javascript
- Library: React
- Location: country-state-city dependency
- React - Select
- React - Toggle
- Fetch: axios dependency
- Test: Postman
Our Journey:
After we received the low fidelity design handoff, the backend developer started working in the backend to implement an API to store the required fields for submitting salary information. In parallel, frontend developer started working in the frontend to implement web components following the hi-fi mockup design.
For the authentication process to verify contributors, the team made a decision to implement a one-time-authentication using an automatically generated magic link instead of a two-factor authentication. Our backend developer had not had experience implementing this type of authentication, he stepped out to the challenges and explored ways to to implement it.
The dev team had recurring meetings to integrate the backend with the frontend. Finally, we achieved the integration without including the authentication in the first version.
Technical challenges
On the backend side, the most challenging part was to implement the one-time-authentication from scratch. It took time to figure out how to implement it. The process to achieve that was:
- Use a famous gem JWT to generate a token per session
- Researching into how to deploy one-time-authentication with rails
- After finishing the implementation, it worked on the local server, but the problem was in the deployment process. Since we were deploying an API, we needed to make certain adaptations in the servers. We modified the deployment files to make it work on production.
The next challenging part was to integrate that with the frontend to complete the authentication process. Since the backend dev had some prior experience with that process, he was able to support the frontend dev in this regard. The integration between the backend and frontend was a success..
Filtering data was another challenging obstacle our developer team had to overcome. Due to the amount of data points within the table; we needed a way for users to filter through data seamlessly, getting the information as they choose their desired filters. Thus we went with a front -end filtering approach rather than filtering within the back-end then making a get request. To accomplish this we simply attached a filter method to the array we pulled from the back-end. From here we used a variety of conditional statements and array methods to ensure our filter toggles and select options matched our data point values.
Since our project is WIP, front end issues such as page pagination & responsive design as well as form validation still exist though these issues will be ironed out over the coming days.
Currently our app does not have any scaling issues as the number of users we have are small. We will need to further evaluate probable challenges that we would face as the user base grows in size where our back-end and front-end could not support the amount of information being generated.
Product Success Metrics
For the MVP, below are some of the success metrics we would be interested in tracking to assess the overall value our product delivers to our users. This will also be an avenue to get customer feedback and incorporate the learnings in future iterations and product improvement.
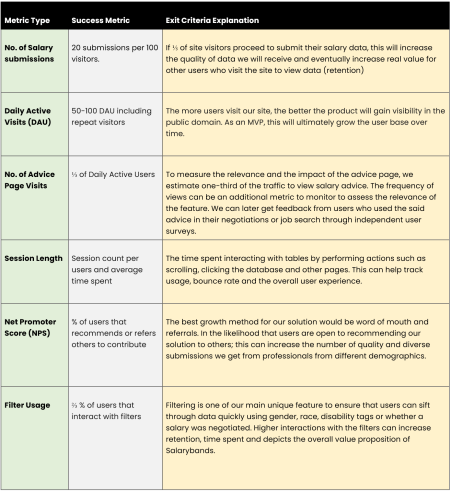
Future Steps
We will be continuing the project with a focus on expanding features as a value add for users to help them level up in their careers. Our team is excited by the opportunity to integrate web analytics, targeted messaging, and automated scraping to retain user anonymity for uploaded documents.
Learnings
Product Manager Learnings:
Robert Appiah
- I learned that your team is your biggest resource. Having a team that is passionate and aligned with the core values and goals of the product you are building is invaluable.
- Working with a cross functional team of software engineers and a designer reinforced the importance of agile teams, lean practices and collaboration when designing innovative products.
- Ruthless prioritization is a superpower of a great PM. A PM has to be able to overlook all the shiny features that could be built and focus on the things that will result in providing the greatest value, user experience and impact for users.
Designer Learnings:
Chriseah Datu
- Performed user research and testing to figure out users’ pain points to build a user-centric solution
- Iterated on design based on changes in product prioritization while working within limitations and constraints discovered in working with developers
- Acquired stronger understanding of best practices for handing-off designs to developers
Designer Learnings:
Jo Sturdivant
- Adapting to an Established Team: Joining the team in week 6 of 8 was challenging, as I had to quickly adapt to existing workflows, dynamics, and goals. This mirrors real-world situations where you often integrate into teams mid-project, and flexibility is essential.
- Work-Blocking for Efficiency: With only two weeks to complete the project, I learned the importance of a structured work-blocking system. This approach allowed me to manage my time effectively and meet deadlines under pressure.
- Making Data-Driven Design Decisions: Unlike my past projects, I had to rely on research conducted by others. This was a valuable experience in using pre-existing data to guide design decisions, helping me focus on the core insights without starting from scratch.
Developer Learnings:
Yersel Hurtado
- Experienced working in a cross functional team
- Understand the importance of focus on specific task
- Work with agile methodology
- Learn new ways of implement authentication process
Developer Learnings:
Vanady Beard
&
As the back-end developer, I learned how important it is to create efficient and reliable systems that support the entire application. This experience also taught me the importance of optimising the database and ensuring the backend is scalable and easy to maintain.
Developer Learnings:
Stephen Asiedu
&
As a back-end developer, I've come to understand the importance of being familiar with various database systems and modules. This knowledge enables me to build diverse applications and maintain versatility in my work. I've also learned that the responsibility for making the right choices rests on my shoulders, guided by my best judgement.
Developer Learnings:
Mackenzie McClemont
&
- Experienced working within a cross functional team and environment
- Worked within an agile environment for the first time
- Learned how to integrate with a back-end via post methods
- Learned how to work with a variety of libraries using their own unique documentation
Developer Learnings:
Maurquise Williams
&
- Process of Creating an MVP: Developing a Minimum Viable Product (MVP) taught me how to focus on delivering core functionalities balancing between essential features and avoiding scope creep.
- Collaboration in a Real-World Tech Setting: This experience taught me how to collaborate efficiently in a fast-paced tech environment, keeping the team aligned and productive, even while working remotely across time zones.
- Sharpening Critical Thinking and Problem-Solving Skills: This experience honed my ability to think critically and solve problems efficiently. By tackling challenges and finding quick solutions, I sharpened my decision-making and troubleshooting skills in a dynamic, real-world setting.
Developer Learnings:
Jeremiah Williams
&
All in all this experience was very awesome I learned that in coding with others being transparent is key
Developers Learnings:
Justin Farley
&
I learned how important communication is when working with a team. Communication provides understanding, advice, ideas, and much more. While working with the product team, I’ve found that communication keeps everything flowing smoothly. Working with a team also showed me that every member brings something different to the table and we all have to work together in order to align and meet our end goal.
Full Team Learning
As a team, some of the key learnings we discovered were the following:
- Team Culture: By setting expectations early, the team was able to increase our odds of success. This enabled us to know what to focus on at each milestone. The PM was able to assess the strengths and weaknesses of each member and found better ways to support, motivate and unblock the team.
- Communication: Our team adopted the mantra “communicate early and often” . With better communication, our team was able to shorten our meeting times but still worked together collaboratively in an agile fashion to show progress and meet deadlines.
- Mentorship: Mentorship matters! We were fortunate to work with Tony who is an experienced engineer from Meta(previously Facebook). Tony served as a sounding board for the team and shared feedback on what we can improve on. The team is grateful for his leadership and advice.