Smarter for You Thermostat
AI-powered thermostat that learns and predicts user behavior for better comfort and energy efficiency.
Product Experience
Problem Statement
How might we simplify smart thermostat usage to maximize energy savings and user comfort?
Problem Background
Smart thermostats were introduced in 2007 with the goal of being easy to use, display energy usage to users leading to energy and cost savings. However, the “Smart” in the name led users to believe that the thermostats would program themselves and automatically reduce energy usage. The “Nest Learning Thermostat” was the first device that responded to user behaviour resulting in direct cost savings. However, research shows that many users find these devices too complex, leading to underutilization of key features and ultimately diminishing the expected energy savings.
Despite being marketed as user-friendly, smart thermostats often have unintuitive menus, complicated setup processes, and features that require manual adjustments. Studies show that smart thermostats can reduce energy consumption by 4-14%, but many users do not experience these savings due to frequent manual interventions and misunderstanding of the automation features. Improper use of smart thermostats has been shown to inadvertently increase energy consumption.
Users need a more intuitive, AI-driven solution that can learn their behaviors and make proactive, rather than reactive, temperature adjustments. Our goal is to bridge this gap by creating a thermostat that enhances user experience while maximizing cost and energy savings.
Research Insights
User Pain Points
- Lack of Awareness: Many users assume that their smart thermostat is already learning their habits when, in reality, they must manually program settings
- Complex Menus: Submenus and multiple settings make it difficult to make quick adjustments
- Energy Consumption Misconceptions: Users do not perceive a significant reduction in energy bills due to manual adjustments disrupting smart scheduling
- Desire for Automation: Users prefer a set-it-and-forget-it experience where the thermostat adapts to their routines
Supporting Data
- 40% of users believe their thermostat is already adjusting based on their habits, yet they manually change settings multiple times per week
- 85% of surveyed users expressed frustration with their thermostat’s complexity
- Users who prefer voice control over menu navigation found current voice commands limited and non-intuitive
Feedback
- “I thought the thermostat was already adjusting for me, but I still have to tweak it every day.” – User Interview
- “There are too many options, and I can never find the setting I need quickly.” – User Interview
The Solution
Key Features & Decisions
- Learned Routines AI: The thermostat automatically adjusts based on learning user behaviors and preferences over time
- Energy Reports & Recommendations: Users receive detailed insights on their energy consumption and personalized suggestions for improvement.
- These suggestions will automatically be implemented unless a user declines to use them.
- Additional Room Monitoring Hardware: Sensors placed throughout the home provide more precise data for room-specific adjustments
- Voice Command Optimization: Improving voice control capabilities to allow users to interact with the thermostat more naturally
Explanation of Solution
During solution explanations, users responded positively to AI-driven automation and energy reporting. By reducing manual input while maintaining comfort, the AI system could significantly improve user satisfaction. Users particularly appreciated the idea of receiving clear notifications explaining why temperature adjustments were made.
User Flow
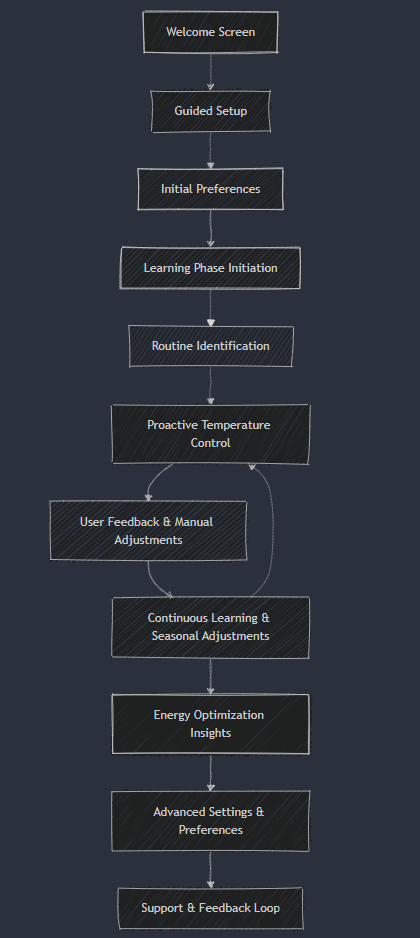
Future Steps
- Further refine AI’s predictive capabilities by leveraging real-world user data
- Expand integrations with third-party smart home ecosystems
- Develop an educational onboarding system to improve user awareness of key features
Potential Challenges & Considerations
- User Trust in AI: Some users may be hesitant to fully rely on AI automation. Building a transparent system that provides explanations for adjustments will be crucial
- Privacy Concerns: Ensuring that data is securely stored and handled with full user consent will be an ongoing priority
Learnings
PM’s Learnings
- User Education is Key: Many frustrations stem from a lack of awareness of existing features. Future smart home products should prioritize clear onboarding processes.
- Communicating backend improvements: Even non-user-facing updates should be highlighted to reinforce trust and demonstrate continuous product enhancement.
- AI Trust Takes Time: While users appreciate automation, they need reassurance that the system is working in their best interest. Providing clear feedback and manual override options builds confidence.
- Prioritization Through Research: Using frameworks like MoSCoW helped focus on high-impact features that address the most critical user pain points. It is difficult to use prioritization frameworks like RICE when working as a sole PM since there is no engineering team to advise on the technical challenges.
Conclusion
The Smarter for You Thermostat project highlights the importance of understanding user behavior when developing AI-powered solutions. By focusing on automation, usability, and energy efficiency, we can create a thermostat that not only enhances comfort but also helps users save money and reduce their carbon footprint. This experience has reinforced the value of user-centered design, iterative testing, and data-driven prioritization in product development.
This document serves as a comprehensive summary of the project, detailing the problem space, research insights, key decisions, and future considerations. Continuous iteration based on user feedback will cement this thermostat’s position as a leader in smart home innovation.
Learnings
Product Manager Learnings:
Viswajit Kumar
Designer Learnings:
Designer Learnings:
Jo Sturdivant
- Adapting to an Established Team: Joining the team in week 6 of 8 was challenging, as I had to quickly adapt to existing workflows, dynamics, and goals. This mirrors real-world situations where you often integrate into teams mid-project, and flexibility is essential.
- Work-Blocking for Efficiency: With only two weeks to complete the project, I learned the importance of a structured work-blocking system. This approach allowed me to manage my time effectively and meet deadlines under pressure.
- Making Data-Driven Design Decisions: Unlike my past projects, I had to rely on research conducted by others. This was a valuable experience in using pre-existing data to guide design decisions, helping me focus on the core insights without starting from scratch.
Developer Learnings:
Developer Learnings:
Vanady Beard
&
As the back-end developer, I learned how important it is to create efficient and reliable systems that support the entire application. This experience also taught me the importance of optimising the database and ensuring the backend is scalable and easy to maintain.
Developer Learnings:
Stephen Asiedu
&
As a back-end developer, I've come to understand the importance of being familiar with various database systems and modules. This knowledge enables me to build diverse applications and maintain versatility in my work. I've also learned that the responsibility for making the right choices rests on my shoulders, guided by my best judgement.
Developer Learnings:
&
Developer Learnings:
Maurquise Williams
&
- Process of Creating an MVP: Developing a Minimum Viable Product (MVP) taught me how to focus on delivering core functionalities balancing between essential features and avoiding scope creep.
- Collaboration in a Real-World Tech Setting: This experience taught me how to collaborate efficiently in a fast-paced tech environment, keeping the team aligned and productive, even while working remotely across time zones.
- Sharpening Critical Thinking and Problem-Solving Skills: This experience honed my ability to think critically and solve problems efficiently. By tackling challenges and finding quick solutions, I sharpened my decision-making and troubleshooting skills in a dynamic, real-world setting.
Developer Learnings:
Jeremiah Williams
&
All in all this experience was very awesome I learned that in coding with others being transparent is key
Developers Learnings:
Justin Farley
&
I learned how important communication is when working with a team. Communication provides understanding, advice, ideas, and much more. While working with the product team, I’ve found that communication keeps everything flowing smoothly. Working with a team also showed me that every member brings something different to the table and we all have to work together in order to align and meet our end goal.