ScreenShopper
An AI assistant that helps PrimeVideo content viewers identify items worn on films and shows, provides options of where to purchase and offers affordable alternatives available directly on Amazon. Enhancing viewer experience, increasing revenue for the streamer and providing greater visibility for retailers/brands.
Problem Statement
How might we identify outfits on shows and provide information on where to shop, enhancing the value of a PrimeVideo subscription?
Problem Background
This inquiry was borne of my own frustration as an avid consumer of TV content, as well as my work as a costume designer; people often wanted to know where certain looks had been sourced from. When people watch shows and films, the primary motivation is entertainment, but this content also inspires viewers. This knock-on effect has led to a billion-dollar product placement industry. In 2023, “film-integrated product placement reached 3.5 billion dollars” with studies finding that “placement in movies can increase sales by up to 47%” and “63% of viewers were more inclined to purchase a product after seeing it placed in a TV show or movie.” However, for fashion specifically, there is a gap, viewers do not have an easy and efficient way to identify the outfits or where to purchase them, without disrupting their viewing experience.
Research Insights
User Pain Points
While I had personal and anecdotal evidence of the problem, I wanted quantitative and qualitative data to assess whether it was widespread enough to warrant a solution. I conducted a survey focused on the shopping and viewing habits of potential users. I also did some social listening on Reddit threads and other social media platforms. Some common pain points were difficulty finding the exact items, inaccurate results, a time-consuming discovery process and regional availability.
Supporting Data
I found that 100% of respondents subscribed to at least one streaming platform, which they watched daily and 70% of them were inspired to buy outfits seen on TV and actively searched for the items. All respondents indicated they would be interested in an effective solution.
Feedback
Through social listening, I found that viewers were frustrated by the “long wait for updates” and “ignored requests” from current solutions for outfit details in particular shows.
Additionally, research surfaced another interesting insight, the need for affordable alternatives, as sometimes people found the exact item but it was outside their budget.
Landing on the Solution
The research validated my personal experience and from the user pain points, I knew the primary challenge was the wasted time spent trying to accurately identify the items and searching for where to shop. However, I also needed a solution that would not detract from the viewing experience of the TV show/film. Also, while a solution could later be applicable to various platforms, I decided to narrow my focus and start with one specific platform- PrimeVideo.
No more waiting for a website to manually tag an item or requests for a specific item from a TV show going unanswered. ScreenShopper can be applied to all content on PrimeVideo and constantly refresh results, so even viewers watching a show months or years after it originally aired, have up-to-date results. It would basically eliminate the lag times and inaccuracies involved with current solutions like websites, reverse image search and social media accounts.
Explanation of Solution
ScreenShopper will identify all wearable fashion in a particular scene, with shoppable links. The viewer will have the option to view by character or by scene. The interface will be similar to how X-Ray is on PrimeVideo, with the viewer able to click on the ScreenShopper icon by toggling on screen or pausing their show/film.
ScreenShopper will be AI-powered, enabling continuous cataloguing of items, so even when the show/film is years old, the item and/or alternatives can still be found. It will be available for all content on the platform, eliminating the need for manual search by viewers and selective information provided by current solutions based on personal
interest or popularity.
User Flows

Link to Prototype
Mockup/Shareable Image
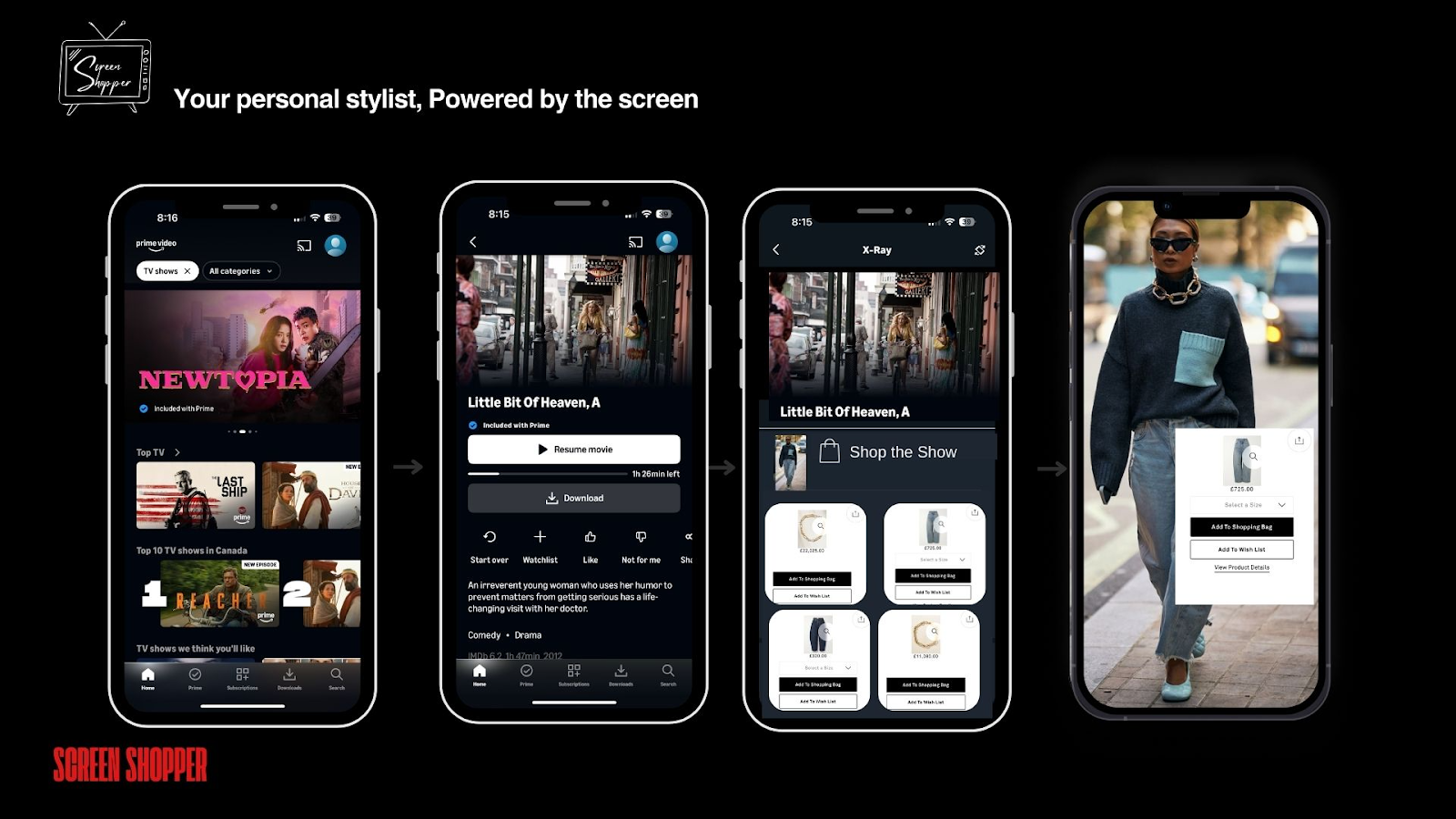
Future Steps
I believe if executed properly this product has broad application and commercial appeal and I am interested in further developing it. When the necessary resources are available, I will be looking to connect with designers and engineers.
Learnings
Product Manager Learnings:
Ivie Ogiemwonyi
This has been an invaluable experience. I learnt about so many ways to leverage AI, new programs and apps to up-skill, how to think problem-first and conduct unbiased research. Furthermore, through the lectures and mentors, it was evident that a PM must keep learning and growing and as I look back at my week 1 submission I can see my growth over the past few weeks. I am excited to implement this mindset as I continue on my PM journey.
Designer Learnings:
Designer Learnings:
Jo Sturdivant
- Adapting to an Established Team: Joining the team in week 6 of 8 was challenging, as I had to quickly adapt to existing workflows, dynamics, and goals. This mirrors real-world situations where you often integrate into teams mid-project, and flexibility is essential.
- Work-Blocking for Efficiency: With only two weeks to complete the project, I learned the importance of a structured work-blocking system. This approach allowed me to manage my time effectively and meet deadlines under pressure.
- Making Data-Driven Design Decisions: Unlike my past projects, I had to rely on research conducted by others. This was a valuable experience in using pre-existing data to guide design decisions, helping me focus on the core insights without starting from scratch.
Developer Learnings:
Developer Learnings:
Vanady Beard
&
As the back-end developer, I learned how important it is to create efficient and reliable systems that support the entire application. This experience also taught me the importance of optimising the database and ensuring the backend is scalable and easy to maintain.
Developer Learnings:
Stephen Asiedu
&
As a back-end developer, I've come to understand the importance of being familiar with various database systems and modules. This knowledge enables me to build diverse applications and maintain versatility in my work. I've also learned that the responsibility for making the right choices rests on my shoulders, guided by my best judgement.
Developer Learnings:
&
Developer Learnings:
Maurquise Williams
&
- Process of Creating an MVP: Developing a Minimum Viable Product (MVP) taught me how to focus on delivering core functionalities balancing between essential features and avoiding scope creep.
- Collaboration in a Real-World Tech Setting: This experience taught me how to collaborate efficiently in a fast-paced tech environment, keeping the team aligned and productive, even while working remotely across time zones.
- Sharpening Critical Thinking and Problem-Solving Skills: This experience honed my ability to think critically and solve problems efficiently. By tackling challenges and finding quick solutions, I sharpened my decision-making and troubleshooting skills in a dynamic, real-world setting.
Developer Learnings:
Jeremiah Williams
&
All in all this experience was very awesome I learned that in coding with others being transparent is key
Developers Learnings:
Justin Farley
&
I learned how important communication is when working with a team. Communication provides understanding, advice, ideas, and much more. While working with the product team, I’ve found that communication keeps everything flowing smoothly. Working with a team also showed me that every member brings something different to the table and we all have to work together in order to align and meet our end goal.